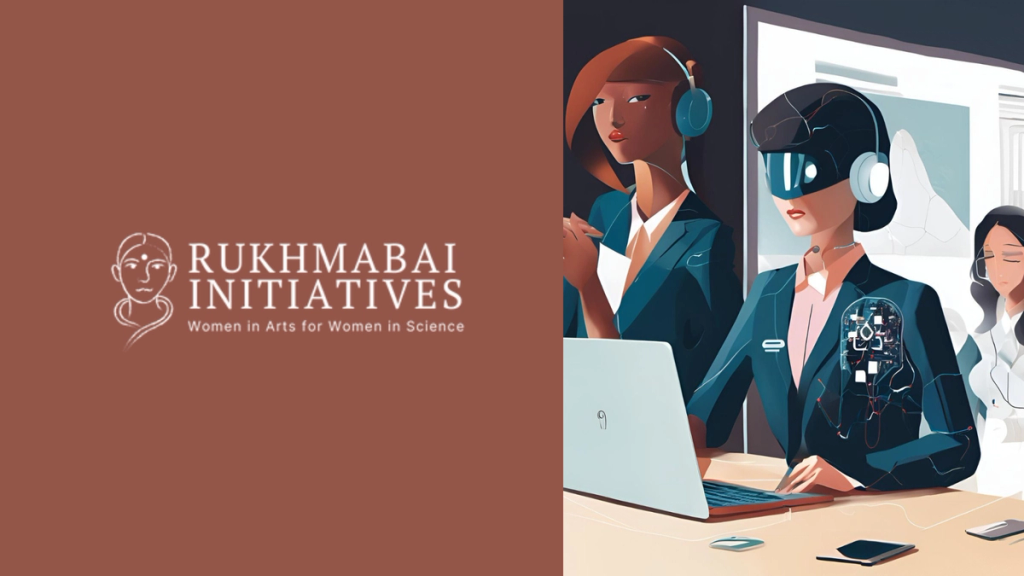
When AI models reinforce gender disparities, women rewrite the algorithm
Senior Research Manager, Aarushi Gupta was featured in this article looking at gender biases in LLMs, the perils and promises of AI and the pervasiveness of bias in technology, and the way ahead.
Technically speaking, the lifecycle of AI development is riddled with elements that perpetuate bias, says Aarushi Gupta, a research manager at Digital Futures Lab, a research network focusing on the interaction between technology and society. Her analysis of LLM use in critical social sectors such as sexual and reproductive health (SRH) and gender-based violence highlights systemic shortcomings emerging at various stages of the process. A major issue lies in the selection of pre-trained base models like GPT, Llama, or Claude; typically, these are chosen for their accuracy and cost-effectiveness, with limited consideration for inherent biases. Biases become more pronounced during customisation — for example, when an SRH chatbot model is supplemented by data from medical journals or government guidelines (through retrieval-augmented generation), it may inadvertently:
- underrepresent women, if the training data is laden with bias;
- amplify sampling biases, like excluding women from poorer or rural backgrounds;
- amplify biases in translation due to inherent cultural or linguistic assumptions. Here, word embedding models may incorrectly assign gender when translating from naturally gender-neutral (like English) to grammatically gendered languages (like Hindi); and
- cause annotation bias, as data is classified reflecting the assumptions of its annotators. In contexts like GBV, for instance, what constitutes harmful or abusive content is interpreted subjectively, leading to a lack of consensus among annotators, and subsequent failure of the AI model to correctly address harmful gender-based content.
Bias permeates in the evaluation stage of AI models too — gender disaggregated approaches to assessments are challenging to conduct because gender biases often manifest in subtle and context-dependent ways, and may not be universally recognised. Gupta also highlights the lack of benchmarking datasets specifically for the Indian population, which are crucial for assessing AI models’ accuracy, fairness and bias, and help operationalise gender bias evaluation better.