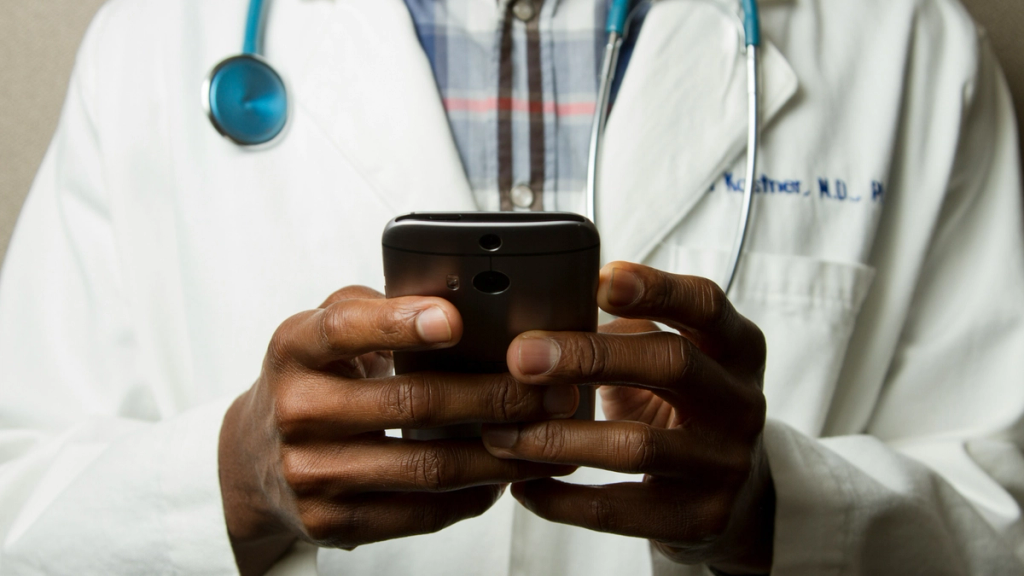
Whose risk it it anyway? Frameworks for Responsible AI in Indian Healthcare
AI is rapidly transforming healthcare in India, but are we prepared for the risks it brings? In this blog post, we introduce From Principles to Practice: Responsible AI in Indian Healthcare, Digital Futures Lab’s latest initiative in collaboration with the Centre for Responsible AI (CeRAI) at IIT Madras. Together, we’re building a context-aware, actionable framework to identify and mitigate the risks of AI across India’s healthcare ecosystem. Learn more about the project from this snapshot.
Artificial Intelligence (AI) is rapidly integrating with health systems worldwide. From helping doctors make clinical decisions to discovering new drugs, AI tools promise to transform clinical care, public health, and research. In India, healthcare faces staff shortages, rural-urban gaps, and persistent financing challenges. AI can help improve service delivery by automating administrative tasks and supporting health workers with tools like screening systems.
But alongside its promises, AI also brings serious risks. These include bias in decision-making, lack of transparency (the "black box" problem), and threats to data privacy. Mistakes such as recommending the wrong drug or missing a tumour in a scan can cause significant harm. This makes AI's safe and responsible use in healthcare more urgent and complex.
The need for a risk assessment framework
Healthcare AI risks vary according to the use case. For instance, if groups defined by race, gender, or geography are absent from an AI screening tool’s training data, it may overlook their health risks or provide inaccurate results, leading to delayed or inadequate care for such groups. At an individual level, AI usage may contribute to medical errors that affect the patient's physical and mental health and well-being.
Additionally, AI risks manifest differently for actors in healthcare systems, such as healthcare providers/clinicians, patients, and hospital management. For instance, healthcare providers face liability risks as they remain responsible for patient outcomes, patients encounter potential health risks, and hospital management must navigate cybersecurity threats linked to handling and sharing sensitive personal data with providers that provide AI tools and services.
Identifying these key risk elements is the first step in mitigating the potential harms AI systems may cause. A risk assessment framework for AI, which identifies key considerations of risks while developing or using AI in healthcare, can be a valuable tool for managing risks at different levels:
- At a hospital administration level, it can assist in making decisions on procuring and using AI.
- At the healthcare provider level, it can be valuable to clinicians using AI or considering its integration as part of their clinical workflows.
- At the level of technology developers and providers, it can guide best practices to minimise downstream risks and ensure compliance with Responsible AI (RAI) principles.
Gaps in existing risk assessment frameworks
Over the past few years, various principles and frameworks have been proposed to guide the development and deployment of AI tools. Multiple institutions, such as academia, the private sector, and governmental and intergovernmental organisations, have proposed AI principles and frameworks. These include the Artificial Intelligence Risk Management Framework by NIST, the OECD AI principles, ICMR’s Ethical Guidelines for Application of Artificial Intelligence in Biomedical Research and Healthcare, and the WHO Guidance on Ethics and Governance of AI in Health.
Currently available risk assessment frameworks can be broadly classified into two types:
- Sector agnostic risk assessment frameworks that focus on the nature and lifecycle of AI but do not consider the sectoral context, and
- Risk classifications based on specific parameters such as outcomes (e.g. risk based on patient harms) or process/components (e.g. data, model, development, and deployment).
While these frameworks are valuable starting points, they lack procedural clarity and don’t address real-world implementation challenges such as identifying, measuring, or addressing risks, recognising the incentives and constraints such as limited budgets, staff capacity, or the need to balance innovation with patient safety.
In particular, these frameworks lack:
- Practical tools for end users, such as doctors, hospitals, or AI developers, to assess risks in their day-to-day decisions.
- A structured approach to risk stratification and mitigation that fits the Indian healthcare context, with its unique challenges and institutional realities.
Without a contextually relevant and structured approach to risk stratification and mitigation, it remains challenging for healthcare providers, clinicians and technology developers to meaningfully interpret, operationalise, and enforce RAI principles in healthcare.
Our approach
We are developing a practical, context-sensitive framework for assessing and managing AI-related risks to support RAI in Indian healthcare.
Our research is grounded in the belief that principles alone are insufficient and that stakeholders need usable tools that reflect real-world constraints and decisions. The framework we are building will help healthcare providers, hospitals and developers identify, map, and stratify risks across diverse AI use cases in the Indian healthcare system. It will also outline clear, actionable strategies to anticipate and mitigate those risks.
We structure our enquiry along the following research questions:
- What are the key emerging applications of AI within the Indian healthcare system? Who are the primary stakeholders in developing, deploying, and regulating such tools?
- What are the key risks and harms associated with emerging AI healthcare tools, and how do these risks vary across different use cases and actors?
- What are the various actionable pathways to ensure safe and responsible AI development and deployment in the Indian healthcare system? Who are the key responsible actors, and how can they be incentivised and supported to adopt and enforce these measures effectively?
Using AI-based tools such as patient flow management systems, screening applications, and personalised care through chatbots as illustrative use cases, our methodology includes:
- Reviewing existing AI risk frameworks and global evidence on AI-related healthcare risks and creating a use-case-based, contextual risk assessment tool,
- Analysing real-world case studies of AI-enabled healthcare tools to validate the risk assessment framework, and
- Co-develop feasible mitigation strategies with stakeholder consultations across the Indian healthcare ecosystem based on practical insights and concerns.
The outcome of this work will be a decision-making tool that enables stakeholders to assess AI-based healthcare interventions in India based on risks and benefits available starting February 2026. This tool will support informed decisions, promote safer implementation of AI technologies, and strengthen institutional capacity for responsible AI adoption in healthcare.
Please watch this space for more updates or subscribe to our newsletter to stay updated.